Series
Data and analytics
Data is essential for every product. These essays will help you improve your skills in analytics and avoid data mistakes.
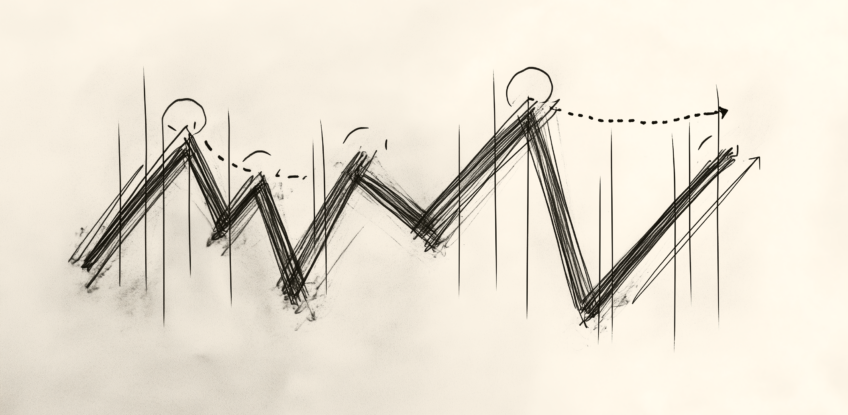
Сreating marketing analytics dashboards the right way: a guide
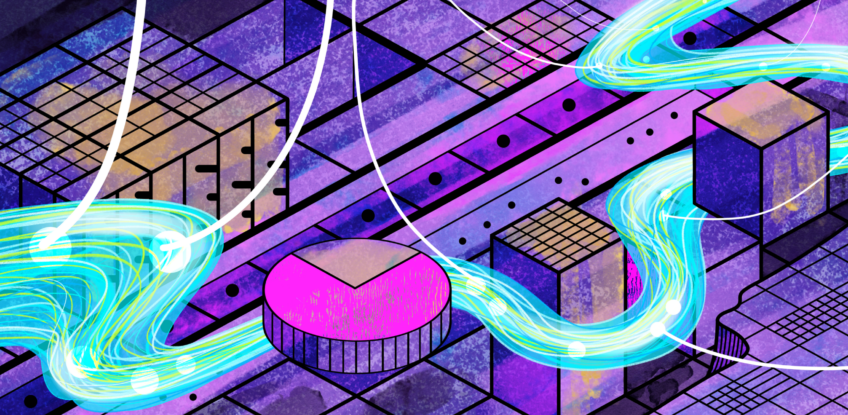
The product manager’s guide to statistical analysis