Retention rate is one of the fundamental metrics in product management. We all use it regularly, yet few of us know that there are many different ways to calculate retention rate. And it is very important to know which one to use when you’re making decisions based on retention data.
Let me start with a story. When I worked at Zeptolab (popular game development company, creator of Cut the Rope, King of Thieves, CATS) once we got an email from a gamedev studio that wanted us to publish their game. We were getting many similar emails, but that one got our attention. We were impressed by the metrics of the game, which had just recently soft launched. According to the developers, Day 1 retention rate of the game was over 55%, and Day 7 retention rate was over 25%.
However, when we started playing and testing the game, something felt wrong. The gameplay was not engaging enough to justify >55% Day 1 retention rate. And the meta game design was not good enough to users in the longer term.
Further investigation revealed that what this game development company called retention was actually “rolling retention.” Classic Day N retention of the game happened to be unimpressive.
This is just one example of how retention metrics can misguide you. There are many nuances in how you can calculate it.
→ Test your product management and data skills with this free Growth Skills Assessment Test.
→ Learn data-driven product management in Simulator by GoPractice.
→ Learn growth and realize the maximum potential of your product in Product Growth Simulator.
→ Learn to apply generative AI to create products and automate processes in Generative AI for Product Managers – Mini Simulator.
→ Learn AI/ML through practice by completing four projects around the most common AI problems in AI/ML Simulator for Product Managers.
Classic Day N retention vs rolling retention
Definition of classic Day N retention and rolling retention
Day N retention shows the percent of users who return to the app on a specified day after their first launch.
Rolling retention shows the percentage of users who return to the app on a specified day or any day after that.
If Day 2 retention is 50%, it means that 50% of new users launched the app on day 2.
If Day 2 rolling retention is 50%, it means that 50% of new users returned to the app on day 2 or later.
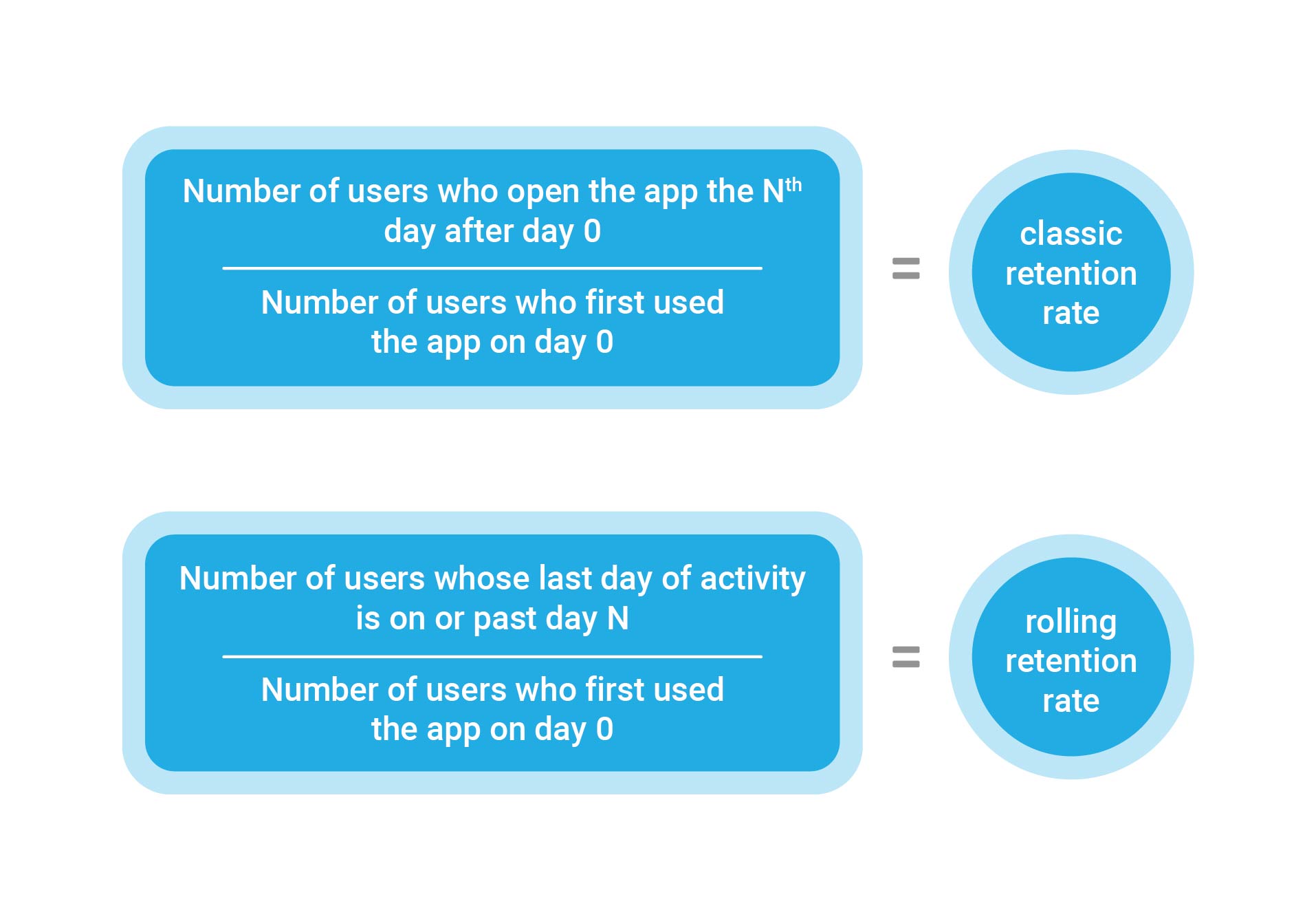