In a series of essays for GoPractice blog, Zakhar Stashevsky, CMO at Letyshops, will explore how to influence growth through effective channel management. Letyshops is a cashback service that allows users to return part of the money they spend on online shopping.
In the first essay, Zakhar discusses why you pay careful attention to the details of attribution model and incrementality when calculating the unit economics (ROI) of your ad campaigns.
Calculating unit economics is easy when you have perfect tracking and attribution for your ad campaigns. But in the real world, perfect tracking and attribution is virtually impossible.
Without understanding the features of the attribution methods used, the specifics of the channels, and the problem of traffic incrementality, unit economy calculation can lead to one of two errors:
- The team underestimates the channel and does not take a full advantage of it
- The team overestimates the channel (this is called incrementality) and loses money
Many teams make these mistakes. We made them ourselves when we were scaling Letyshops. Thanks to this experience, I can now share my thoughts on how to identify and avoid these errors.
→ Test your product management and data skills with this free Growth Skills Assessment Test.
→ Learn data-driven product management in Simulator by GoPractice.
→ Learn growth and realize the maximum potential of your product in Product Growth Simulator.
→ Learn to apply generative AI to create products and automate processes in Generative AI for Product Managers – Mini Simulator.
→ Learn AI/ML through practice by completing four projects around the most common AI problems in AI/ML Simulator for Product Managers.
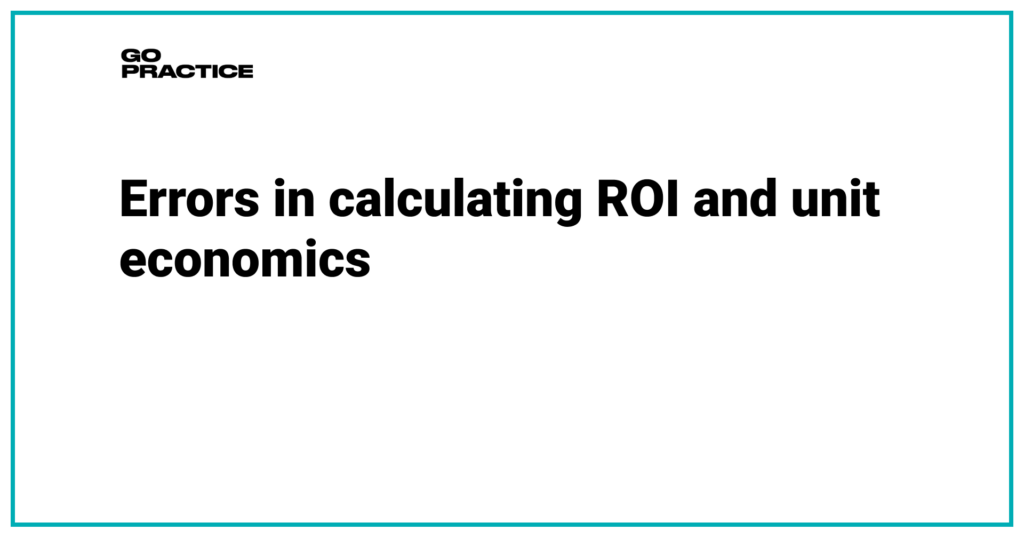
Two common errors in calculating the unit economics
An attribution model is a set of rules and technical decisions based on which you determine the channel an attracted user or sale should be assigned to.
To assess the objective effectiveness of a channel, it is important to understand exactly how the selected attribution model works for your product and the advertising channel.
Each set of “channel + product + attribution model” has its own important characteristics. Many teams don’t realize this, which leads to errors in assessing the effectiveness of the channel (i.e., calculating the ROI and unit economics).
Different attribution models will affect the accuracy of evaluating the effectiveness of advertising channels for certain products in different ways. In some cases, they will lead to an underestimation of the channel, and in others, an overestimation. Both will result in money loss.
Overestimating channel efficiency (aka incrementality)
The problem of overestimating the efficiency of a channel is often called the “problem of incrementality.”
In this situation, you attribute targeted actions (installs, purchases) to the advertising campaign but in reality, they would have occurred without the campaign in question.
Let’s take a look at a simple example. Say I want a Big Mac and head to the nearest McDonald’s. On the way, I see a Big Mac poster ad. The ad doesn’t affect me because I already decided to buy a Big Mac. In a digital setting, my Big Mac purchase would be attributed to this ad. Because of this, when analyzing the advertising channel, its effectiveness would be overestimated, which can lead to a loss of money when using it.
Underestimating channel effectiveness
Most marketers are afraid to overestimate the effectiveness of the channel, because it leads to money loss. But underestimating the channel is no less dangerous. It may also lead to money loss, albeit indirectly.
Channel underestimation occurs when some of the users who came as a result of advertising are not attributed to their corresponding campaign. Because of this, the channel will seem to be less effective than it actually is.
This leads to incorrect calculation of the ROI and, as a result, unaggressive scaling of the channel or even the refusal to use it. Consequently, the team misses growth opportunities and opens up loopholes for competitors.
How underestimating the channel leads to missed opportunities
At a certain point in the work on Letyshops, we achieved product/market fit. Letyshops is a cashback service that allows you to return part of the money spent when shopping online. The product effectively solved the problem of saving while shopping, which ensured good retention of users.
We found an effective growth channel through YouTube bloggers. The first tests showed that the unit economics of the channel was converging (ROI was positive): we got back the investment made in advertising in the six-month horizon.
So we assigned the marketing team the task of scaling the channel with the following restrictions:
- ROI > 0;
- The payback period is less than six months
YouTube channels became one of the main growth drivers for Letyshops and delivered excellent results. But after a while, we realized that due to the peculiarities of the attribution model, we had made mistakes in assessing the effectiveness of the channel. And this prevented us from using its potential to the fullest.
In retrospect, it is difficult to assess the scale of the opportunities we missed. But it was huge. If we hadn’t made mistakes in assessing the channel’s effectiveness, then today the word “cashback” would be associated with Letyshops instead of banks or mobile operators. We were among the first companies to create such a product, but we failed to fully realize the opportunity we had.
How the specificity of the attribution model hindered the channel’s potential
As we began to actively advertise through YouTube influencers, Letyshops began to grow rapidly.
YouTube channels were perfect for our product and solved several problems simultaneously:
- YouTubers onboarded users into the product at the ad level. And they did it better than our welcome materials and other ad sources.
- YouTubers presented the essence of the business model in a very accessible way. In 2016, it was difficult to explain what cashback is and where this money comes from.
- The recommendation model reduced product distrust. The blogger shared the social capital with our brand, thereby lowering the threshold for entering the product.
The unit economics of the Youtube channel was great. All we had to do was to hire people for the team and scale the channel. Alas, the euphoria did not last long.
As the channel scaled, its efficiency began to decline. As we used more and more YouTubers, the relevance of new ad campaigns gradually declined. The key limiting factor was the requirement for a six-month payback period. Growing volumes with this limitation became more and more difficult.
We had been looking for solutions for a long time in approaches to the implementation of ad campaigns and the creation of new creatives, experiments with new channels. But the growth opportunity was actually in a completely different dimension.
The problem was how we measured channel performance using our attribution model. By default, we evaluated the results of ad campaigns in isolation from other channels and used the Last Paid Click attribution model based on UTM tags. As a result, most users who came through advertisements from a Youtube blogger were not attributed to the advertising campaign (many users didn’t click the ad link and just directly went to the website), and the ROI of the channel looked worse than it actually was.
Because of this, we halted promotions through YouTubers when, based on our unit economics calculations, they stopped to meet the required criteria. But as we later found out, most of these campaigns matched these criteria. We just miscalculated the efficiency of the channel.
How did we notice the problem of underestimating the channel efficiency when calculating the unit economics
The problem with the quality of the attribution model became apparent when we began to analyze the number of new users who came organically.
With high-quality and effective attribution, the number of new organic users becomes quite predictable—there isn’t much volatility in this channel in the absence of obvious external factors (i.e., announcement, PR).
But our graph looked different. It became clear that users who come from other channels were not properly attributed and disguised themselves as organic traffic.
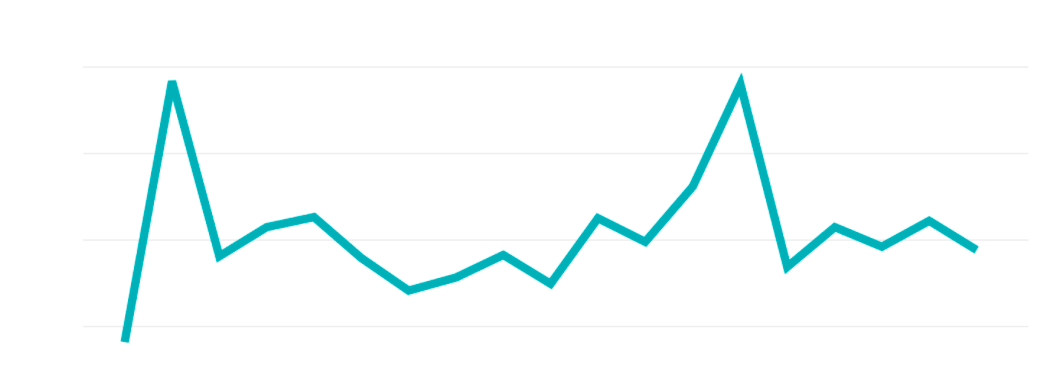
Image: Sign-ups in Letyshops (organic users)
But even after realizing the problem, we couldn’t quickly calculate the objective value of the YouTube blogger channel. We tried different attribution models, but we couldn’t pinpoint the correct factor.
At the time, other large channels were also working in parallel, making it difficult to separately assess their impact on organic traffic. Moreover, promotions from bloggers on YouTube have a long time effect (people continue to watch videos long after their release), which makes it difficult to evaluate the effectiveness of new campaigns.
The solution was to run an isolated experiment in another region, which helped determine the correction factor. After that, we began to multiply the results of advertising campaigns tracked by advertising links.
The coefficient turned out to be significantly greater than 1. This means that we could be many times more aggressive in purchasing ads in the channel. Of course, we began to use this new knowledge, but the window of opportunity had already been closed.
Window of opportunity in the channel—or why you should aggressively use unprotected growth channels
While we were looking for a real correction factor, the competition in the channel had increased.
Indirect competitors emerged and started purchasing ads from YouTubers. This affected its cost and, as a result, the amount of traffic that we could buy.
Soon, direct competitors appeared in the channel, which not only fought for advertising inventory, but also competed at product level, which reduced the strength of our product/market fit.
Even though we were the first to find this channel and make it work for our product, our ineffective attribution models and incorrect ROI calculations caused us to refrain from using it to its full potential. The possibilities are finite, so you are either a fast startup or a dead startup.
How underestimation happens, and how to avoid it
The problem of underestimating advertising channels comes up in the following situations.
Promotion of products with a long sales cycle. For example, if you are developing a complex SaaS product, people may interact with the product ads many times as a result of marketing across different channels. But when using Last Click attribution, all value will be attributed to the last ad touch, or even added to the organic traffic record.
Cross-device gap. For example, a user saw an ad of your app on YouTube from a desktop computer and followed the advertising link to a landing page on the web. Everything is fine and the UTM tags were registered in the database. But instead of clicking on the install link in the landing page, the user went directly to the app store and installed it from there. As a result, the user will be attributed to the mobile organic channel, not the YouTube channel.
Big marketing mix. In this case, some of the channels may be overestimated, and some may be underestimated, which will lead to incorrect allocation of budgets.
What are the sources of underestimation problems?
Usually, the problem of underestimating effectiveness comes up in channels where there is no explicit call to click on an advertising link or the channel is an intermediate step in a long sales chain:
- TV advertising
- Radio advertising
- Advertising on YouTube channels
- Podcast advertising
- Display advertising with delayed effect.
- Content marketing
- PPC + content marketing
- Content Marketing + SEO
- PPC + Sales Team
- Content Marketing + Sales Team
When using these channels, you should pay attention to organic traffic. Often, other channels are disguised as “organic” users.
It is also worth paying attention to the way channels work in combination with each other. If you launch display ads in combination with other performance channels and see an improvement in the results of the latter, then you should consider revising the effectiveness of that channel.
Solution: selecting optimal attribution models for each channel
A common mistake many teams make is trying to find a single attribution model that works across all channels. But there are no silver bullets.
There is no single attribution model that can help you measure the effectiveness of all channels. Their specificity is very different. A more efficient approach is to develop solutions for individual traffic channels.
Below are high-level descriptions of possible ways to measure attribution for different channels. We will look at them in more detail in the next essay of the series.
What approaches can be used for different channels:
- Facebook, Instagram, Criteo: You can use built-in attribution models in ad managers, but you must also conduct tests to measure the incrementality of ad channels to avoid overestimating their effectiveness ( we will talk more about this in the next part of the essay);
- Advertising using bloggers on YouTube, Instagram or TikTok: Promotional codes or individual landing pages, spike analysis, questionnaires after the targeted action, launching tests in different countries/geographical locations;
- TV ads: Spike analysis, Brand awareness, post-target action questionnaires;
- Email marketing: A/B tests with a selected group.
In addition to the attribution models mentioned above, there are algorithmic and probabilistic models. We will discuss them in more detail in a future essay.
It is worth remembering that each of the proposed methods has its own pros and cons, so it is worth choosing a solution that also matches the specifics of your product.
How channel overestimation (aka incrementality) causes money loss
Above, I discussed how underestimating a channel can lead to lost opportunities and money. Here, I want to show how the opposite—overestimating the effectiveness of a growth channel—can also be detrimental to your marketing strategy. In this case, some of the users who were attributed to a specific channel came from another source.
In such situations, marketers talk about the problem of “incrementality of advertising campaigns”—how much of the received users precisely came because of advertising.
An example of the incrementality problem when buying paid search ads for your brand
Almost every product buys paid search ads for its brand. This is usually done because competitors like to spin on brand queries. Sometimes webmasters do it, if you have an affiliate program, reselling your organic traffic to you.
In the classical calculation of the unit economics, the ROI of such campaigns is very good; the most targeted traffic comes from brand queries. But if you make decisions based on these numbers, then there is a great chance of losing money.
Maybe you just pay for those who would have come anyway by clicking on the first link in organic search? Or could a competitor buy ads and affect the users’ behavior with ad placement?
This can be verified with a “checkerboard”.
Using “checkerboard” to assess the incrementality of buying paid ads for branded queries
Approaches to assessing incrementality vary across channels. But in the case of buying a branded context, there is a simple and effective method called “checkerboard.”
The essence of this method is to turn on the ads one day and turn them off the next. The same sequence is repeated over a month. Sometimes a different time interval is used, for example, an hour.
A month after starting the checkerboard procedure, you count the number of new users who came organically and from brand paid ads in dynamics by day. The difference between the results on the days when the ad was running and on the days when it was not will show the actual number of additional users as a result of such an ad.
Such an exercise will most likely show that the real ROI of PPC advertising per brand is significantly lower than what you see with the “correct” calculation of the ROI and unit economics. You’ll likely find it beneficial to turn off this channel or leave the “checkerboard” on permanently to spend less on advertising and at the same time monitor the situation on brand search queries, as it may change.
The problem of overpricing ad purchases for branded queries is very insidious. Sometimes, such queries get mixed with other ad campaigns and because of them, these campaigns look much better than they actually are. Therefore, it is worth separating the purchase of brand context into separate campaigns and carefully assessing their incrementality.
Which channels are most susceptible to the incrementality problem and problem-solving methods
The problem of channel incrementality is most acute in situations where you use many advertising channels or you have a strong brand.
When using a large marketing mix on large budgets, one user can be attributed to several channels at once. This effect is called overlap. If the difference between the actual profit that you have and the amount of profit attributed to different sources exceeds 10-15%, then it is worth starting to investigate this problem.
Channel overestimation often occurs with strong brand products. In such situation, a large number of people know about your product and decide to use it at some point before being exposed to your ads. If you are actively using ads, then there is a high probability of accidentally exposing users who would have used your product anyway to the ads. In this case, all the merits for acquiring a user will be given to a random advertising channel, however, this channel didn’t have any real impact on these users.
Which channels are most susceptible to the problem of overvaluation (incrementality)
Channels with large reach are prone to the problem of incrementality, where it is possible to accidentally hook a user who was already ready to buy or start using a product. This is usually (but not limited to):
- Advertising in online cinemas, which must be watched to access the content;
- Ads on Facebook, Instagram, and pre-rolls on YouTube using Post View attribution. On Facebook, you just need to see an ad in your feed to be attributed to your campaign using the Post View attribution model.
- Clickunders are a commonly used channel. It is found on content resources that have limited ways to monetize. Example: you “google” information that interests you, go to the site from the search results, but, in addition to the site itself, you open another tab with the advertiser’s site.
- In-App traffic: advertising in free apps, where you need to view ads to get access to the functionality or get other bonuses (in-game currency);
- Remarketing: this channel often has a small incrementality, as it targets an audience that already knows about your product or has previously used it, but stopped using it for some reason. That being said, the likelihood is high that these users will organically return. But with the right approach and working with segments, this tool can be quite effective.
- Email marketing is an almost free advertising channel for businesses. It is often used in a chain with others. In case of incorrect analysis, the email marketing campaign can “drag” results onto itself, which will affect the objectivity of the channel’s effectiveness assessment.
The problem of incrementality and fraud
The problem of incrementality gives rise to many opportunities for fraud and deception.
Unscrupulous partners can launch contextual ads on the brand and put their referral link, reselling your organic traffic to you.
An unscrupulous agency could run a Facebook ad campaign to maximize reach and use Post View Attribution. Their goal is to catch the maximum number of people who would install an app or purchase the product anyway, but attribute this result to themselves by showing them ads before taking the targeted action. The incrementality of such a campaign will be minimal, although the unit economics can converge perfectly and ROI will look great.
Fraud and deception masked by the incrementality problem manifests itself in a large number of variations in different channels.
Approaches to assessing the incrementality of advertising campaigns and solving the problem of channel revaluation
Below are high-level descriptions of possible solutions to the problem for different channels. We will consider them in more detail in the next essay of the series.
The solutions to the channel overestimation problem are similar to those suggested for the underestimation problem.
- Run incrementality tests in ad networks where possible (Facebook, Criteo)
- Launch advertising to a part of the audience with an isolated control group
- Launch advertising in a separate region, which can then be compared to a similar region (in terms of behavior) where there was no advertising
- Turn advertising channels on and off in order to observe changes in the overall result and the result of individual channels (taking into account seasonality and product changes)
- Use algorithmic attribution models (Markov chains, Shapley vectors)
- Use probabilistic attribution models
There are more ways to solve the channel overestimating problem than the ones I mentioned above, but it is important to understand what is right for your product. It is worth remembering that complexity doesn’t necessarily increase the effectiveness of a channel. We will consider examples of such cases in other essays.
Key conclusions: Unit economics, attribution methods, and channel incrementality cannot be considered in isolation
In a world with perfect attribution, calculating unit economics and ROI is easy. But that doesn’t happen in the real world. The performance of attribution models will vary across different products and channels.
Therefore, it is impossible to consider the unit economics and ROI calculation separately from the attribution model, the model’s efficiency and channel incrementality.
Some teams don’t know which attribution model they are working with and they simply use the default provided by their analytics system. Others choose without diligence and therefore have no idea how effective this model is in different growth channels. Others choose wisely but don’t change the model and they way how they use it as the product and marketing mix changes.
Different attribution models have their pros and cons, are suitable for different channels, and have different impacts on the problem of traffic incrementality and misattribution. The attribution models we selected in Letyshops only partially helped to see the value of the channels, which prevented the correct calculation of YouTube’s ROI. This severely limited the team and resulted in a smaller window of opportunity.
In this essay, we examined the problems of underestimating or overestimating the advertising channel when calculating the ROI due to not taking into account the attribution and incrementality model.
In the next essay, we will discuss in more detail how these problems can be solved for different channels and products by selecting effective attribution models and assessing the incrementality of advertising campaigns.