As more companies aspire to be data-driven, the role of the data analyst is becoming crucial both to the organization and to product managers themselves. In fact, the World Economic Forum found that the data analyst/scientist role had the most increase in demand in 2020. Clearly these positions are incredibly needed.
What does a data analyst do? A data analyst is responsible for gathering, organizing, and interpreting data to provide business insight. Typically this insight is used to solve an issue, make a decision, or determine performance. Simply put, a data analyst interprets data to drive better business outcomes, which is exactly why product managers must collaborate with them effectively.
Data analysts may perform specialized roles, like product analyst, performance analyst, or customer insights analyst. The term data analyst is often used interchangeably with data scientist, though data scientists are typically more focused on predicting business outcomes using techniques like machine learning. An emerging role is the data product manager, who taps into data to support all traditional product management activities. And any of these roles may work with a data leader, whose job is to oversee all data projects in the organization.
To get more information on how product managers can work well with these roles, we asked industry experts the following questions:
- How should responsibilities be split between product manager and data analyst?
- What are some examples of ineffective collaboration you’ve seen between product managers and data analysts?
- What are the best practices for product managers and analysts to work well together?
- Would your answers for the above questions change based on the stage of the company, e.g. startup vs. blue chip?
The answers led to incredibly helpful advice that you can use to enhance data analysis in your team right now. We’d like to thank this great group of data-minded product leaders for sharing their experiences with us:
- Gavin Deadman (Lead Product Manager, Betfair at Flutter Entertainment Plc.)
- Luka Gligić (Data Scientist at Meta)
- Ankit Goswami (Senior Manager of Product Management – Global Ecommerce Checkout at Expedia Group)
- Diego Granados (Senior Product Manager at LinkedIn)
- Varun Kalyan (Senior Product Manager, Marriott International)
- Cyrus Poteat (Product Manager at Collectors Universe, ex Zillow)
- Sanant SK. (Data Product Manager at Vista)
- Marcelo Savignano (Lead Product Analyst at Miro)
Keep reading for their guidance on getting the most out of your team’s collaboration between product management and data analysts.
Q: How should responsibilities be split between product manager and data analyst?
While the product manager and data analyst roles are distinct, there is certainly overlap. This means that deep collaboration is needed to be effective. Product managers define strategy and how the product will implement and deliver on that strategy, but data analysts determine how to answer the questions that help product managers achieve their mission.
Product managers need to have data analysis skills to perform ad hoc analysis, communicate with data analysts effectively, and understand the analysis results. Data analysts need to understand the product context so that they can apply the appropriate statistical techniques to create the insights that product managers need. Data analysts help product managers dig into their hypotheses and explain the results they are seeing.
Alignment is critical between the two roles. Including data analysts as part of the product team and sprint planning is most effective. At some organizations, data analysts may be part of a centralized team. While this can work, it’s generally not as beneficial as embedding the analysts in the product team. A centralized model may encourage more of a services approach, where the analysts only respond to requests rather than taking ownership of solving product questions.
Varun Kalyan (Senior Product Manager, Marriott International)
In my perspective, product managers ideally should possess data skills to make the first and second level analysis and gather insights. This makes the product manager a very well-rounded individual who can communicate using data and visualizations.
Depending on the organization, there are data analysts placed at a squad level, which is amazing if available, as the person is directly in sync with the team’s cadence and schedules and product roadmap. Leveraging this person’s data skills is paramount to being a successful growth-driven product manager.
Sanant SK. (Data Product Manager at Vista)
Product managers should have a “data sense” – understand how the customer journey is captured through various data points, gain knowledge on the “why” behind the various tools that help in translating the data into decision, and have a learning mindset to the techniques used by the analyst in order to collaborate well.
I personally like the framework of a data mesh as given below (which is followed at Vista where I work):
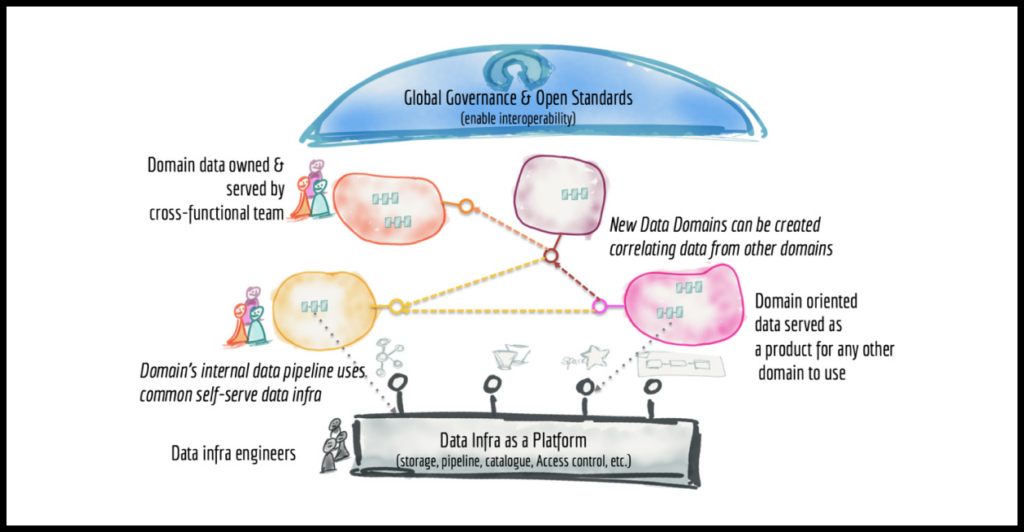
The analysts are present both in data domains (data domains are formed based on the part of the business where they are focused) as part of a cross functional team where they are involved in long term projects as well as building self-service data products. There are line of business aligned analysts too in order to answer ad-hoc and strategic questions directly from business stakeholders.
Ankit Goswami (Senior Manager of Product Management – Global Ecommerce Checkout at Expedia Group)
Product managers are responsible to define product vision, strategy, roadmap, and champion cross functional product development efforts. They start by defining the why and what of the product with active customer interaction and market opportunity based on the inputs from data analysts.
Data analysis can be described as the process of applying statistical and mathematical techniques to better illustrate data. Data analysts’ primary focus lies in:
- Performing strategic and ad-hoc data work in support of product managers.
- Identifying opportunities in data to accelerate product adoption, retention, engagement, and/or monetization.
- Developing dashboards and defining metrics that inform success for the product team.
- Helping design, execute, and evaluate A/B tests to improve the user journey.
- Exploring large, complex, and loosely defined datasets to create actionable insights.
- Explaining trends across data sources, potential opportunities for growth or improvement, and data caveats for descriptive, diagnostic, predictive (including forecasting), and prescriptive data projects.
Their key skills are centered around conducting research, written communication, critical thinking and tech skills (SQL, R, Python programming, spreadsheets, etc.).
Luka Gligić (Data Scientist at Meta)
In my experience, this collaboration works best when the roles are divided but not separate. What this means is that at all times there should be complete alignment between the product manager and the data scientist on the goal, in a way that even when working in isolation their efforts complement each other.
In terms of analysts being part of the product team vs. a centralized data team, I had a radical change of thought throughout my career, starting from the latter but now firmly convinced of the former. The reason for this is that centralised data teams can sometimes be understood as a service function that works mostly reactively on requests from product managers. This limits the data scientists from having a full grasp of the problem and applying the best solution. Sitting with the product team allows data scientists to develop area expertise and better ensure the success of the product.
Marcelo Savignano (Lead Product Analyst at Miro)
In my experience, the best collaboration between product managers and analysts usually happens when there is a shared passion for improving products, and the firm conviction that learning from users is the best way to improve products in a meaningful way.
For a PM, the main questions to answer are:
- How can I make my product better?
- How can I make it more successful?
- How can I reach and delight more users?
- How can I make their lives better?
For analysts, it’s important to help the product managers dig deeper into these high-level questions, and come to the point where an analysis can be carried out. For example, if the product manager has learned that some users complain about a particular feature, questions the analyst could help answer are:
- How often is this problem occurring in real life?
- What type of users are affected by this problem?
- Is it really an issue we need to prioritize right now, or are there more pressing issues to solve?
Q: What are some examples of ineffective collaboration you’ve seen between product managers and data analysts?
Collaboration between product managers and data analysts is vital to a successful product. However, our experts have seen mistakes negatively impact this collaboration that you’ll want to avoid, such as:
- Thinking about data collection and involving data analysts too late in the lifecycle of the product. Products need to be instrumented appropriately with a data plan created from the start so the team will have the data it needs.
- Treating data analysts as a service that queries a database or generates a number for product management. This stifles problem solving.
- Failing to communicate key measurements, goals, or success metrics.
Most of these mistakes are the result of lack of understanding of each role’s responsibilities and the information that needs to be shared. When roles and priorities are clear, things go more smoothly.
Cyrus Poteat (Product Manager at Collectors Universe, ex Zillow)
One big pitfall is not accounting for data needs when defining product requirements. My role at Zillow often revolved around fixing this issue and developing processes that addressed missing or problematic data. Often this occurs when there is a need to stand up processes and solutions quickly. Our approach to the issue was to create clear swim lanes for how each team contributes to identifying data requirements, data contracts, and building the needed pipelines. If the responsibilities aren’t clear in this area, collaboration won’t yield results or won’t be possible.
Diego Granados (Senior Product Manager at LinkedIn)
Some of the worst cases that I’ve seen is when the product manager treats the data analyst like the interface of a database or as a request machine – just submitting tickets and expecting the data without sharing the vision and strategy for the customers. When you don’t give the complete picture or when you don’t involve the data analysts in your processes, they don’t have the context. They might be able to give you some data but that doesn’t mean that it’s the best data that you could use for your product.
Luka Gligić (Data Scientist at Meta)
I would say the most common one I see is using data scientists as a number generator. Very often, others on the team spend time thinking about an idea and then decide what number they would need to justify it. While this can work sometimes, it is not something they should be spending their time on.
Instead of asking for a number (e.g. can I get the percentage of users that open our application each day and report an issue?), the best way is to ask a data scientist the question behind it, like can we understand the reliability of our application in a data driven way? This way, the cross-functional team has identified a possible issue (reliability), but leaves it open to the data scientist on what is the best way to answer it.
Sanant SK. (Data Product Manager at Vista)
I asked my team of analysts this question and most of the answers below have been shared from them.
We should never use the data analyst as a substitute for the interface of a database. This is why product managers should know how to deal with data. Also, complete autonomy sometimes leads the analyst down a rabbit hole due to the interesting nature of insight generation.
Not understanding each other’s perspective is another pitfall. Product managers and analysts see things differently, have different skill sets, and often slightly different goals. While an analyst will always try to “dig deeper” to deliver a (near) perfect solution, a product manager might want to find the “most feasible solution” given the real-life constraints. It’s essential for both parties to have an understanding of each other’s goals.
Asking the analyst for output instead of outcome is another mistake. When given the proper context, analysts are often able to quickly point out data insufficiencies or alternative sources of data which can result in a better solution.
Q: What are the best practices for product managers and analysts to work well together?
While avoiding mistakes is a good defensive move, a product manager would be wise to proactively invest in their relationships with data analysts. Teamwork between these two roles determines the success of any data-driven project, ultimately resulting in great products with happy customers in the best circumstances.
To make sure the liaison flourishes, product managers need to embed the data analyst as part of the product team. Regular communication is of utmost importance, as is reducing friction through clear understanding of shared goals. The data analysts should be given ownership over data requirements and also the freedom to experiment and produce novel insights.
And of course product managers should be using the data analysts’ work to guide product direction and decision making from the very beginning of the project. Product managers should be ready to change direction according to the data, while data analysts should find the data that is actually needed to answer the right questions.
Luka Gligić (Data Scientist at Meta)
The key is regular communication. I speak to my product manager almost every day and have several dedicated meetings. This allows for ideas and feedback to be shared early and regularly ensuring full alignment along the way and preventing sunk cost fallacy issues.
Key advice for product managers here would be to use data from the start to guide product direction, and not the other way around, while the data scientists need to plan their work based on the needs of the product, and not the other way around.
Gavin Deadman (Lead Product Manager, Betfair at Flutter Entertainment Plc.)
An effective way of a product manager working with analysts is collaborating with them on the entire product delivery lifecycle – identifying problems/opportunities, validating solutions with customers before build, and measuring the impact of the product iteration once live. Work closely as one team.
Cyrus Poteat (Product Manager at Collectors Universe, ex Zillow)
Conducting sprints where analysts can plan their work alongside the rest of the team in coordination with the product manager works well for an established team. This can be combined with an iterative approach where the analyst can mock up data structures or visualizations in response to requirements, get feedback, and then iterate from there.
The most effective data analyst should seek to own the data as fully as the product manager owns the product. This level of ownership looks like taking in requirements and defining data and metrics from top to bottom. This isn’t common as there are often fewer analysts than product managers, but I think product teams should aspire to this.
Ankit Goswami (Senior Manager of Product Management – Global Ecommerce Checkout at Expedia Group)
When we say product managers need to be data informed, the first step to data literacy is to build a better relationship with data analysts. From ideation, the product requirements document, execution, and release, collaboration with data teams can help build deeper confidence for product decisions.
Encouraging tighter feedback loops with data analysts leads to faster time to iteration of product ideas. Product managers can move forward with their product decisions confidently. The analytics team can collaborate with the product team to conduct user research, create cohorts, and test feature functionality and product usability. The partnership with analytics enables the product team to experiment with multiple feature options so they can ship a feature that matches user needs and improves the product experience.
Diego Granados (Senior Product Manager at LinkedIn)
Effective ways for me to make these collaborations start with good communication. I want to make sure that my team is constantly informed of what’s going on. If we make a decision they need the strategy, vision, and features. They have to know why.
But communication is just one part. The culture of the team – not the company but the team – is even more important. Because you can’t have a proactive, effective team with good collaboration if somebody is afraid to speak up. The product manager is not in charge of creating that culture, but the product manager is in charge of helping to foster that culture: making sure that everyone feels included, everyone feels safe, everyone feels comfortable, but also that everybody understands what is happening and where the team is heading.
Varun Kalyan (Senior Product Manager, Marriott International)
The most effective way for a product manager and analyst to work together is by defining and refining a healthy backlog for the data team. This helps each team member prioritize their work efficiently and keep the company’s vision, broad product roadmap, and team-level priority front and center. Cross collaboration between product managers and analysts with regular weekly sync ups are best practices to keep the communication channels open always. Weekly meetings help decouple the priority items from the rest and keep the teams aligned and focused.
Working with data analysts to do competitive analytics to see how similar players are doing, what is working, what’s not working, industry benchmarking, and market trends is important. Working in a very structured format increases efficiency across the product and analytics teams.
Q: Would your answers for the above questions change based on the stage of the company, e.g. startup vs. blue chip?
We all know that companies at all stages need to use data to drive decisions, however the circumstances and culture at each individual company will dictate the best way to do so. While some of the answers above apply universally, others should be adapted to the individual situation.
For example, in a startup, product managers must be willing to take on more of the data analysis. There probably aren’t data analysts or scientists available or dedicated to the product team. This responsibility would fall to the product manager. Another difference in a startup is that there may not actually be much product data available to analyze, especially in the early phases. If this is the case, product managers must rely on user, market, and secondary research to help fill the gaps.
In a larger organization, the product manager must focus more on collaboration, communication, and providing clear guidance. The product manager should still have data analysis skills, however this mostly won’t be the main aspect of their job unless they are a data product manager. Product managers in large organizations also need to ensure they are including data analysts as part of their core team.
Also keep in mind that data, along with its insights, can actually be considered its own product. Companies at any stage can benefit from a designated data leader who can ensure that the organization is data-driven.
Gavin Deadman (Lead Product Manager, Betfair at Flutter Entertainment Plc.)
In a startup the analyst (if there is one) would often not have time to support the product manager, so the product manager would be expected to capture their own insights by diving into tools and conducting market analysis, reviewing insights daily, and reporting on performance. In a blue chip company the product manager would have analysts to support them with capturing and analysing data. The product manager would need to focus more on collaboration and having clear strategy and objectives, but still should have access to the systems and know how to pull and review insights as well as conduct market analysis.
Marcelo Savignano (Lead Product Analyst at Miro)
In startups, roles are usually less clear. People wear many hats, and it’s harder to split responsibilities. You may find product managers doing their own analysis (e.g. in the absence of analysts), or analysts conducting their own surveys (e.g. in the absence of researchers).
Another question is whether the data at scale exists. Startups prior to finding product-market fit probably have to rely more on user research as their product has not entered habitual use and therefore data can not be a reliable source to understand user behavior.
Diego Granados (Senior Product Manager at LinkedIn)
If in a startup there are only three people and there are no clear boundaries in the roles, adapt to it. If you have an organization with hundreds of people and there are 50 of them in your team, once again, adapt to your role. It’s more about being conscious of the manpower or resources that you have and the time you have more so than trying to be strict with definitions and boundaries. As long as there’s good understanding, there’s a clear idea of where the team is heading next. As long as there is good collaboration and good communication.
Cyrus Poteat (Product Manager at Collectors Universe, ex Zillow)
There are differences but I think the same principles apply. One important concept is the idea of a data leader. Typically found as data engineering directors or analytics leaders, I find this role only tends to exist in more established businesses. For startups that aspire to be truly data-driven, having a data leader from an early stage should be a high priority. Businesses may think the CTO will handle it, the product leader will handle it, or analysts will handle it, but data is not software. It is a product in itself and requires its own leaders to think holistically about how data should be structured to work for the company.
We’d like to thank Stephanie Walter for incredible help in creating this article.