This is part of a series of articles on activation and making sure users experience and appreciate your product’s value.
In this article, we will discuss how to measure and not measure activation efficiency in a product.
→ Test your product management and data skills with this free Growth Skills Assessment Test.
→ Learn data-driven product management in Simulator by GoPractice.
→ Learn growth and realize the maximum potential of your product in Product Growth Simulator.
→ Learn to apply generative AI to create products and automate processes in Generative AI for Product Managers – Mini Simulator.
→ Learn AI/ML through practice by completing four projects around the most common AI problems in AI/ML Simulator for Product Managers.
All posts from the series
01. When user activation matters and you should focus on it.
02. User activation is one of the key levers for product growth.
03. The dos and don’ts of measuring activation.
04. How “aha moment” and the path to it change depending on the use case.
05. How to find “aha moment”: a qualitative plus quantitative approach.
06. How to determine the conditions necessary for the “aha moment”.
07. Time to value: an important lever for user activation growth.
08. How time to value and product complexity shape user activation.
09. Product-level building blocks for designing activation.
10. When and why to add people to the user activation process.
11. Session analysis: an important tool for designing activation.
12. CJM: from first encounter to the “aha moment”.
13. Designing activation in reverse: value first, acquisition channels last.
14. User activation starts long before sign-up.
15. Value windows: finding when users are ready to benefit from your product.
16. Why objective vs. perceived product value matters for activation.
17. Testing user activation fit for diverse use cases.
18. When to invest in optimizing user onboarding and activation.
19. Optimize user activation by reducing friction and strengthening motivation.
20. Reducing friction, strengthening user motivation: onboarding scenarios and solutions.
21. How to improve user activation by obtaining and leveraging additional user data.
Working on user activation only makes sense after achieving product/market fit. If a product doesn’t add enough value to convince the user to change the way they solve a problem, then even a perfect activation process won’t help.
But after achieving product/market fit, activation becomes one of the main levers that influence product growth. Activation improvement has a non-linear effect on growth and often provides the highest ROI on investment.
Common mistakes when choosing user activation evaluation metrics
Before discussing how to measure the effectiveness of user activation, let’s look at some common mistakes.
Activation score based on the percentage of users who are onboarded
Many teams measure activation performance based on the percentage of users who successfully finish onboarding.
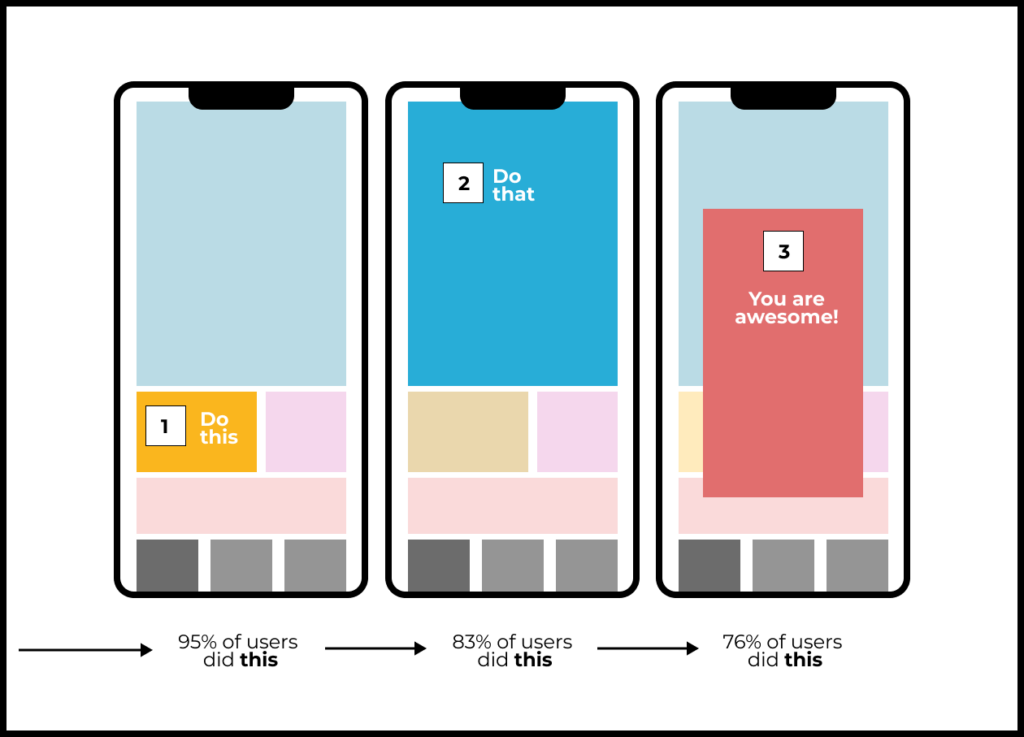
Analyzing the causes of user dropouts at key stages of the onboarding funnel is useful. But optimizing the activation process for this metric alone is misguided.
It is possible to create an onboarding process that has near-100% conversion. For this, it is enough to reduce it to one step. Unfortunately, the effectiveness of conveying value in this case will be close to zero.
Your task is to find the best way that will allow the user to understand how and where the product can be useful to her. This path will inevitably create resistance, but it is necessary to achieve the desired effect.
Assessing activation based on the proportion of users who experienced the “aha moment”
When the user first experiences the added value of the product, they have their “aha moment.”
Many teams define their product’s “aha moment” and then evaluate activation performance based on what proportion of users experienced it. This is a much better approach than the previous one. But in isolation, this metric can also lead to wrong decisions.
First, for many products it is impossible to define a single “aha moment” that applies to all users. A service can solve several different tasks (fulfill different “jobs” in the terminology of the JTBD framework). In this case, the paths to experiencing the value will vary for different use cases. Highlighting a single “aha moment” and its corresponding activation metric will result in the team pushing all users down a single path that doesn’t make sense for everyone.
For example, in Dropbox, new users can come from different channels and with different jobs to be done. Someone might have received a download link from a friend; someone else might want to organize file storage in a team; another might want to free up their smartphone’s memory by uploading some of their photos to the cloud; and maybe someone was referred to the app through a friend.
The user’s shortest path to value will depend on the specific use case. Therefore, each of these use cases will require different “aha moments” along with their corresponding metrics and activation mechanisms.
If the team chooses one “aha moment” and pushes users to it with all its might, this will limit the potential for delivering value to users with other use cases in advance.
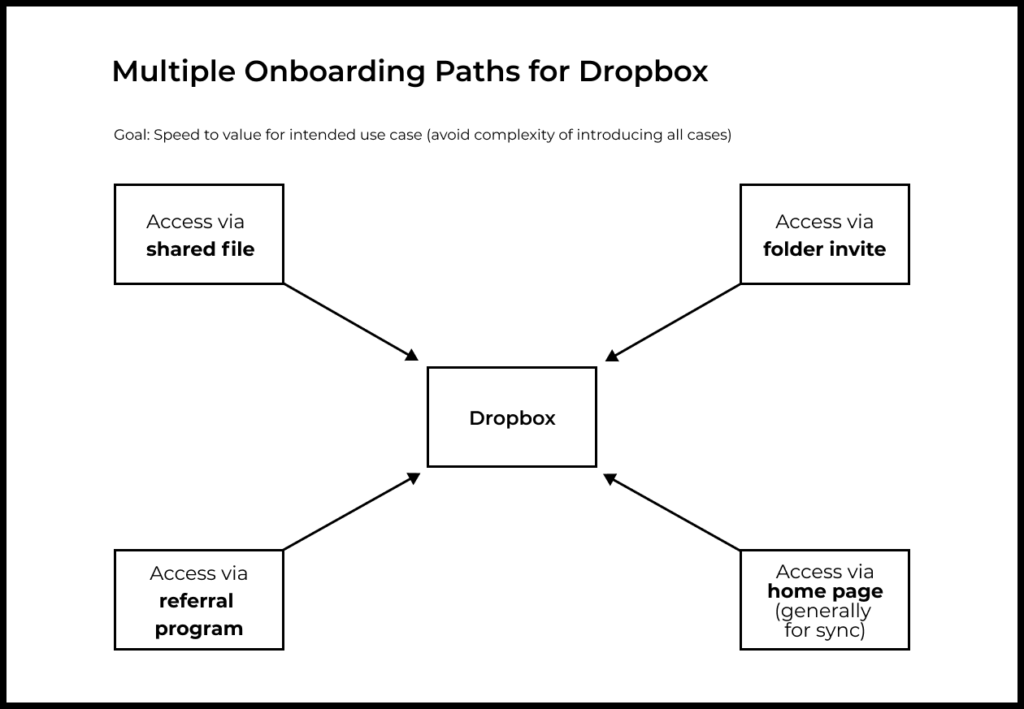
Missing evidence of causal relationship between the chosen activation metric and the long-term user success metric
Another common mistake is that the team fails to verify whether there is a causal relationship between the chosen activation metric and long-term user success.
In order to prove the existence of a causal relationship, the team must conduct an experiment that measures how changes in the activation metric affects user success (e.g., the effect of the number of followers on user retention). If the experiment proves that this metric really affects the long-term success of users, then the team can focus on improving it.
But even then, there are some risks. An attempt to represent the complexity of the world exclusively through metrics inevitably leads to loss of important information.
Assume that Instagram defined its “aha moment” as a situation where a new user follows ten users. Achieving this number increases the likelihood of seeing high-quality content in your feed and getting feedback on your own posts. After that, the team runs an experiment and proves that increasing the proportion of users with ten follows affects long-term retention. Next, the team starts looking for ways to increase the proposed activation metric.
This approach becomes problematic when the team starts thinking in terms of metrics and loses focus on the users behind these metrics.
In reality, there’s a difference between following ten active and close friends, ten inactive acquaintances, ten celebrities, or ten random accounts. But at the metric level, they will all look the same.
Moreover, the team may decide that to improve the activation metric, they can arbitrarily follow ten other accounts on the user’s behalf. This will improve the activation metric, but will not necessarily result in the user experiencing the value of Instagram.
This doesn’t mean that the choice of a metric characterizing the “aha moment” doesn’t make sense. But it is important to know that these metrics should not be the only way to evaluate product decisions related to user activation.
Measure activation performance based on the proportion of users who become regular users of the product
To measure the effectiveness of activation, it is better to use metrics that reflect the proportion of new users who regularly use the product to solve their problems. Meanwhile, instead of one-time usage, you should track how much the product fulfills users’ “jobs.”
For example, if your product solves a regular task, then the effectiveness of activation should be evaluated based on retention on the target action. This is the main signal of successful delivery of the added value to new users.
For an e-commerce marketplace, a good metric for evaluating activation is purchase retention. For a messenger, activation should be measured by retention on sending messages. For a travel app, it is hotel booking.
Metrics that track the volume of certain activity from a cohort are even better: the total volume of purchases on the marketplace (GMV from the user cohort), the total number of messages sent in the messenger, the number of bookings through the travel service. These metrics characterize how much of a person’s “job” your product is fulfilling. The more your product can take away this “job” from alternative solutions, the stronger its product/market fit is.
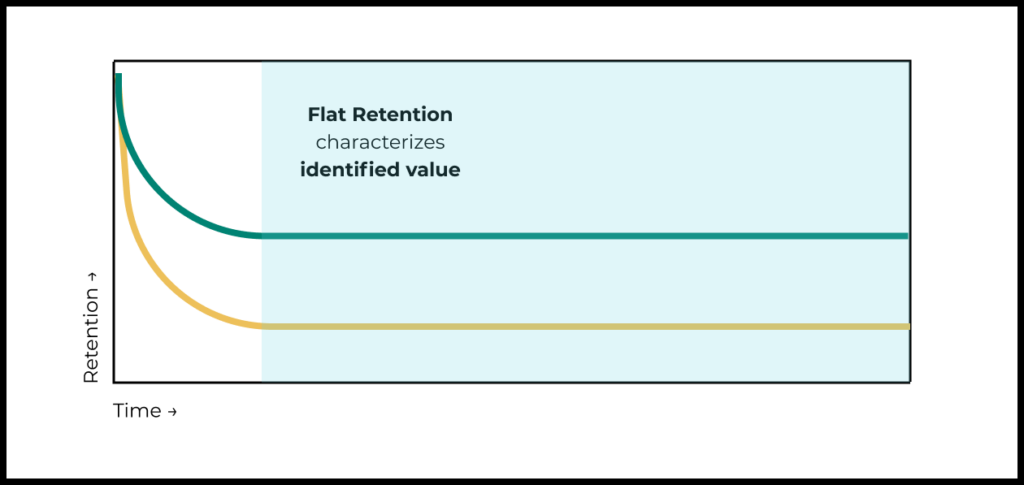
Key steps to user success
Working on activation is aimed at learning how to convey the value of the product to the maximum share of new users from the target market segment.
That is why, to analyze the effectiveness of activation, we evaluate the level at which retention on the target action plateaus, i.e., the amount of “job” that the product fulfills for a new user.
This doesn’t mean that metrics representing the intermediate steps to user success are useless. While they shouldn’t be used to evaluate the effectiveness of activation, they help to understand exactly how different product changes affect the user experience, and how this change translates into retention or other key metrics.
In the next article, we will discuss intermediate metrics that affect user activation. To do this, we must understand how the product creates added value in the context of the problem that the user wants to solve. Based on this, we will unfold the necessary steps to reach success:
- We will learn how to define the “aha moment”—the moment when the user first experienced the value of the product;
- We will learn how to determine the necessary conditions so that the user, in theory, can get value from the product.
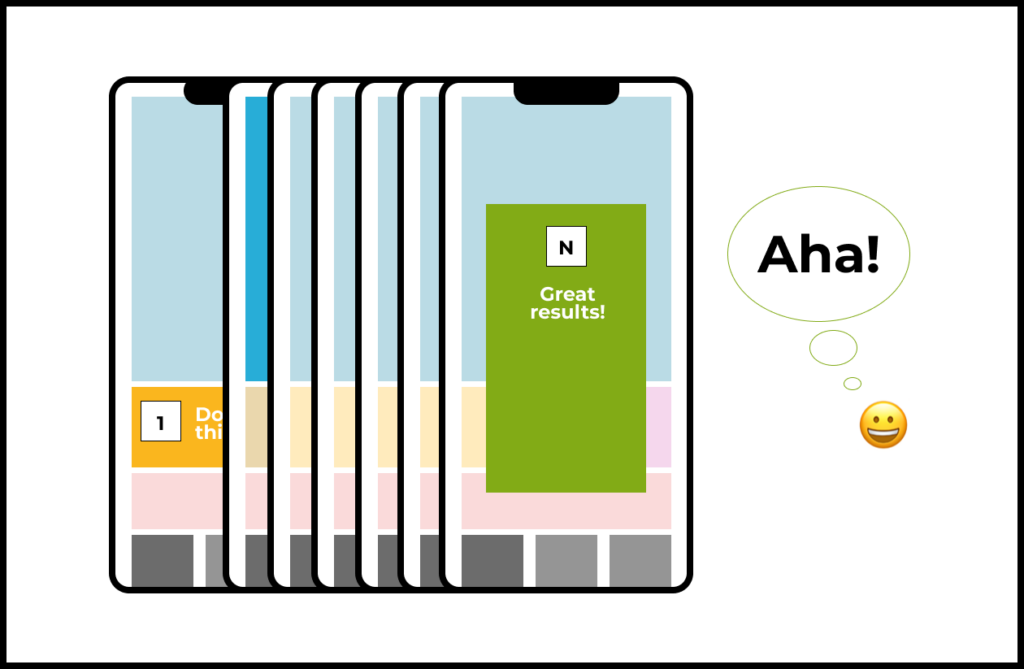